Better context: Multimodal AI analyzes different inputs and recognizes patterns. Thereby, leading to natural and human-like accurate outputs. Accuracy: Since multimodal AI combines different data streams, it can result in more reliable and precise outcomes.
Multimodal AI technology is a groundbreaking approach that enables artificial intelligence systems to process and integrate data from multiple modalities, such as text, images, audio, and video, to produce more accurate and contextually relevant responses. By combining these diverse data inputs, multimodal AI can interpret information much like humans do—by synthesizing context from all available sensory channels. This technology has applications across fields like robotics, healthcare, customer service, entertainment, and more, pushing the boundaries of what AI can achieve.
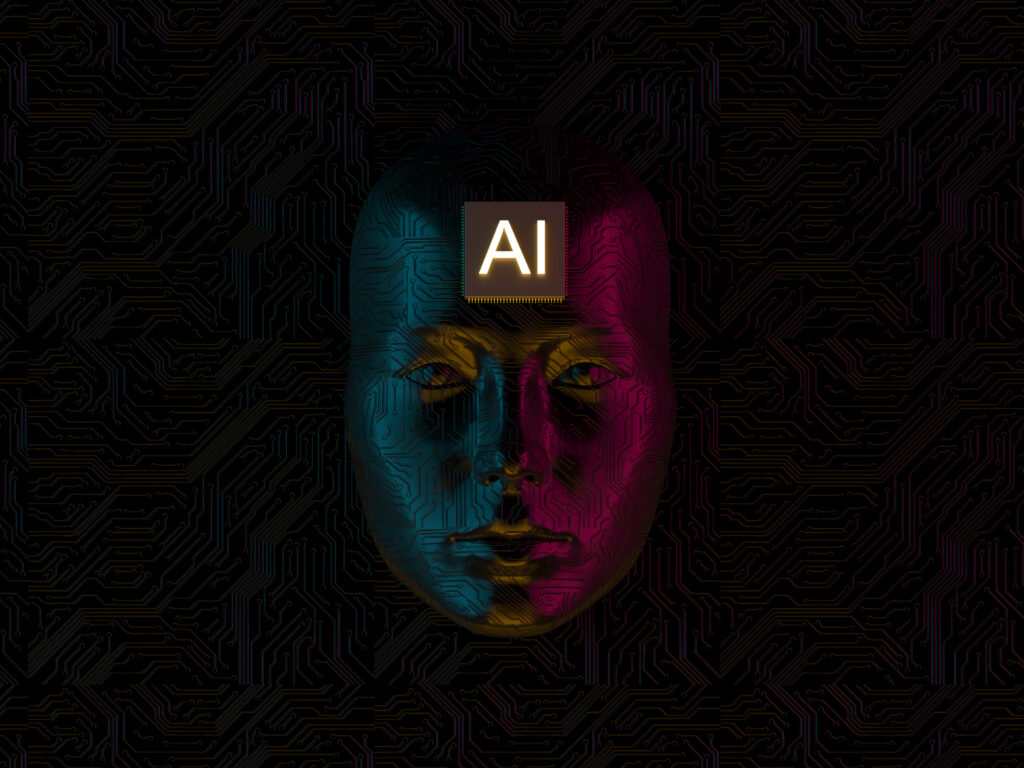
Key Components of Multimodal AI
- Cross-Modal Understanding Multimodal AI can connect data from different sources, understanding how each piece of data (text, image, audio, etc.) relates to the others. For example, in a video, the AI could analyze not just the visual elements but also the spoken content, background sounds, and any accompanying text to generate a comprehensive understanding.
- Feature Alignment and Fusion To process multimodal data, AI models must align and fuse features from each modality. This involves synchronizing elements like spatial patterns in images with temporal cues in audio or associating key phrases in text with relevant visual components. This feature fusion is a complex process that requires advanced neural architectures, such as transformers, to learn the relationships across modalities.
- Contextual Coherence and Reasoning By integrating data from multiple modalities, these models can provide richer, more context-aware responses. For example, in an e-commerce setting, a multimodal AI could help users find products by analyzing text descriptions, images, and even user behavior to offer a more tailored shopping experience.
Technologies & Models Advancing Multimodal AI
- CLIP by OpenAI CLIP (Contrastive Language–Image Pretraining) is a model that learns to associate images with text descriptions, enabling it to perform tasks like identifying objects in images based on a textual query. CLIP represents a significant step in cross-modal learning, allowing for applications in content moderation, image search, and more.
- DALL-E by OpenAI DALL-E is designed to generate images from textual descriptions. By synthesizing visual elements based on natural language prompts, DALL-E showcases how AI can create coherent and contextually relevant images, pushing creative boundaries in fields like design and advertising.
- Flamingo by DeepMind Flamingo is a model designed for image-based question answering, able to interpret images and respond to questions about them. This technology enhances applications such as medical imaging, where a model could assist in diagnosing based on both text and image data.
- Speech2Face and Speech-to-Text Models These models convert spoken audio into text or even attempt to reconstruct facial images based on voice characteristics. By linking audio and visual modalities, such models can be used in biometrics, virtual assistants, and enhanced accessibility tools.
- GPT-4 Multimodal (e.g., ChatGPT) ChatGPT, now with multimodal capabilities, can analyze text and images to generate responses. This enables more interactive experiences where the AI can understand image content in addition to responding to text-based queries, broadening its utility across fields like education, customer service, and content creation.
Applications of Multimodal AI
- Healthcare Multimodal AI can integrate patient records, radiology images, and lab results to support diagnosis and treatment planning. For instance, AI can combine CT scans with clinical notes to predict patient outcomes more accurately.
- Autonomous Driving In autonomous vehicles, multimodal AI integrates data from cameras, LiDAR, radar, and GPS to create a cohesive understanding of the vehicle’s environment, improving safety and decision-making in real-time.
- Customer Service and Virtual Assistants Virtual assistants powered by multimodal AI can understand spoken commands, visual cues, and context to provide more accurate and personalized assistance. This is especially useful in customer service chatbots that handle text, voice, and visual data.
- Education and E-Learning Multimodal AI systems can enhance learning platforms by adapting content based on video, text, and audio data, providing a richer and more interactive educational experience.
- Entertainment and Content Creation Multimodal models like DALL-E and ChatGPT can aid in the creation of multimedia content, generating images, animations, or videos based on text prompts, making it easier for creators to bring their ideas to life.
- Robotics Multimodal AI enables robots to process visual, auditory, and text data to perform complex tasks autonomously. Robots in warehouse settings, for example, use multimodal input to locate, pick, and handle items.
Challenges in Multimodal AI
- Data Integration: Different modalities have unique structures and must be harmonized. Ensuring data is correctly aligned and fused is a significant technical challenge.
- Computational Demand: Processing multiple data types simultaneously requires significant resources, making it demanding for real-time applications.
- Bias and Fairness: Multimodal systems trained on diverse data sources may inherit biases from each modality, necessitating robust bias mitigation techniques.
- Interpretability: Understanding how multimodal models make decisions based on complex, combined data inputs can be challenging, limiting their transparency.
The Future of Multimodal AI
As multimodal AI technology advances, we can expect even more seamless, intuitive, and versatile applications. Multimodal AI will likely be a cornerstone in creating machines that can understand, interpret, and interact with the world in a human-like way, enhancing everything from digital assistants to personalized healthcare and beyond.
Share this content: